
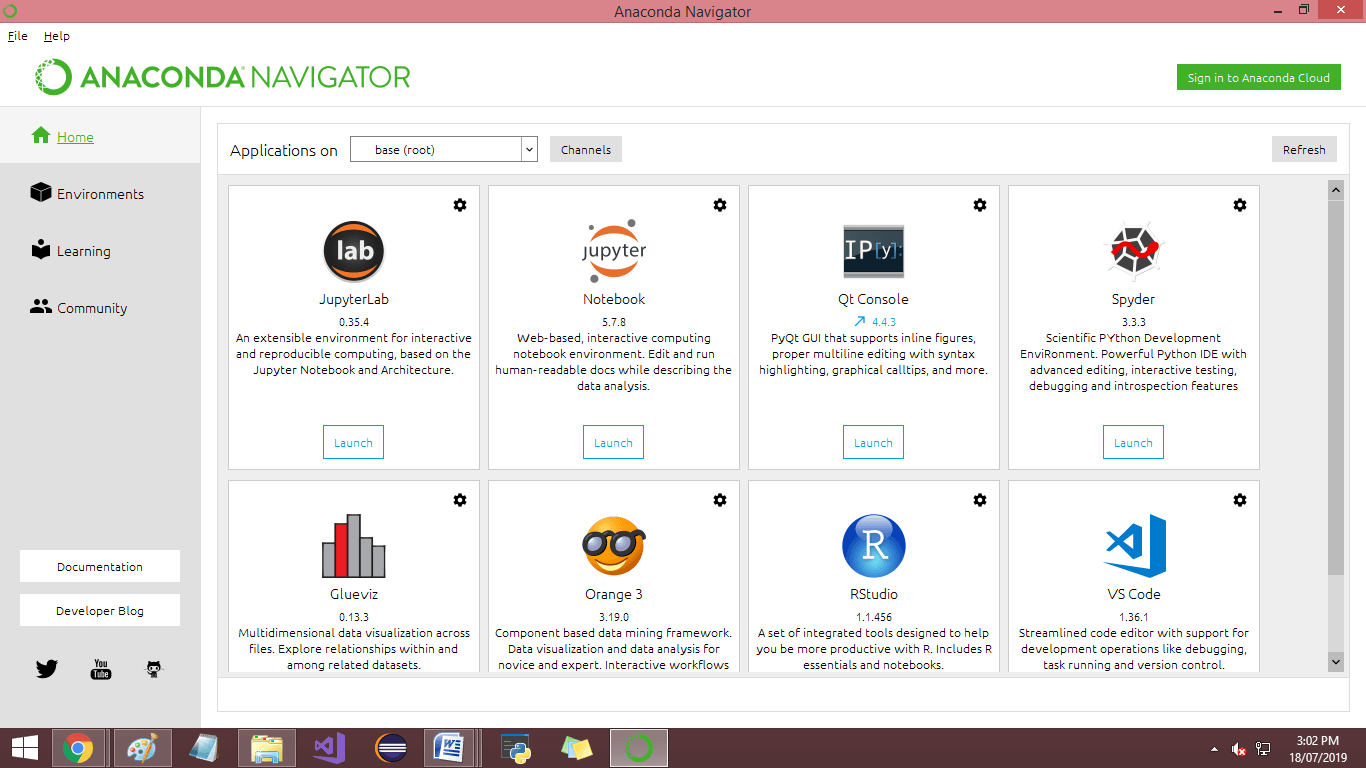
You are definitely better off with a custom distribution than with the vanilla Python.Intel distribution takes these from its own channels. To perform the tests, I activated the distribution (for both Anaconda and Intel distro) and created a virtual environment, and installed all main dependencies using conda – namely sklearn, scipy, numpy and pandas.
ANACONDA VS PYTHON NUMPY FULL
First one is a clean Ubuntu with clean Python 3.6.5, second one was running the Anaconda distribution, and the third had a full Intel Python distribution installed. More about the installation can be found here. Source /opt/intel/intelpython3/bin/activateĬonda create -n intelpython -override-channels -channel intel python=3.6 intelpython scipy pydaal scikit-learn numpy pandas
ANACONDA VS PYTHON NUMPY INSTALL
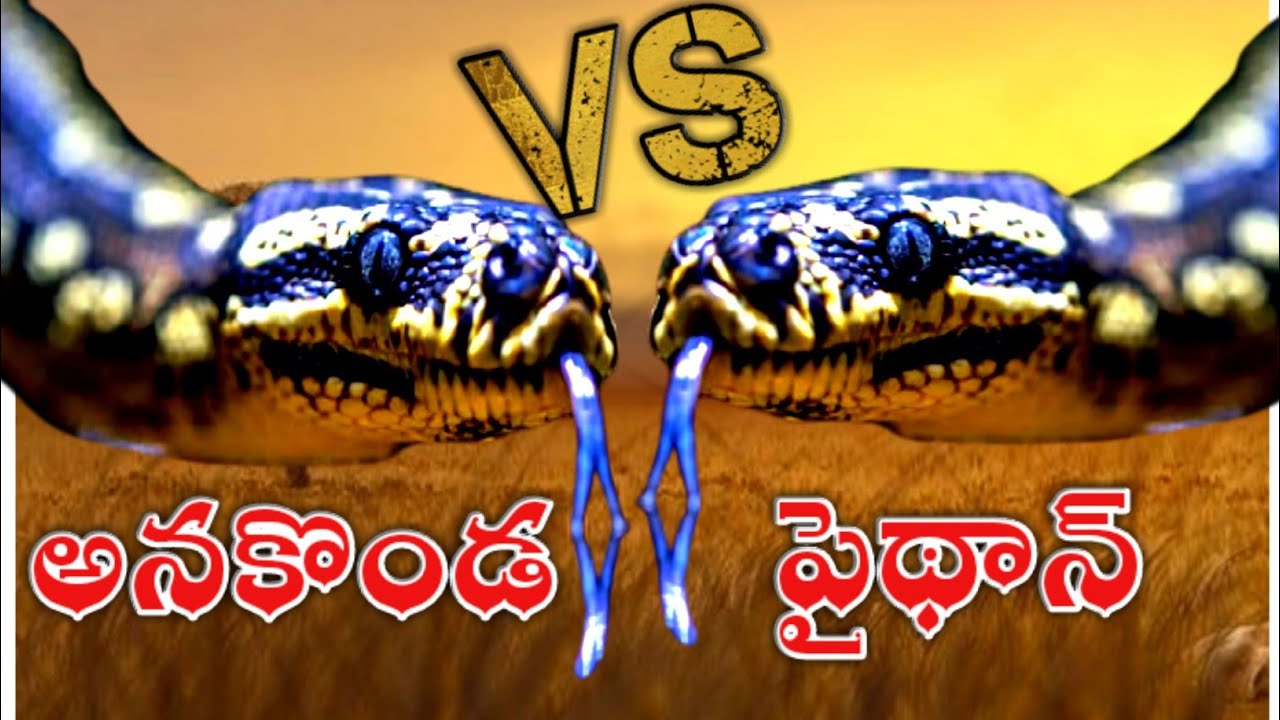

If the CPU is from AMD, the MKL does not use SSE3-SSE4 or AVX1/2 extensions but falls back to SSE no matter whether the AMD CPU supports more efficient SIMD extensions like AVX2 or not. This is because the Intel MKL uses a discriminative CPU Dispatcher that does not use efficient codepath according to SIMD support by the CPU, but based on the result of a vendor string query.

The MKL runs notoriously slow on AMD CPUs for some operations. "However, the numerical lib that comes with many of your packages by default is the Intel MKL. Some highlights since I figure you can click the link to read the entire thing if interested: Permanent solution for Linux: echo 'export MKL_DEBUG_CPU_TYPE=5' > ~/.profile Simply type in a terminal: export MKL_DEBUG_CPU_TYPE=5īefore running your script from the same instance of the terminal. Opening a command prompt (CMD) with admin rights and typing in: setx /M MKL_DEBUG_CPU_TYPE 5ĭoing this will make the change permanent and available to ALL Programs using the MKL on your system until you delete the entry again from the variables. This post from reddit has a much more thorough explanation of what's going on, but it's just a one liner in your terminal to trick MKL into thinking you are an Intel system since MKL does nasty things to non Intel devices:
